Read time: 8 mins
Seasoned researchers understand that maximizing insight quality requires careful planning about the structure of each research project. This planning involves two critical decisions: which research design to implement and which data collection methodology to use. However, the most compelling research strategies often go beyond traditional single-method approaches, instead leveraging multiple mixed methods research design to capture deeper, more nuanced insights. Especially when the subject matter is complex, forward-thinking researchers partner with experienced teams who can facilitate innovative approaches that yield optimal results rather than defaulting to familiar techniques.
Key Takeaways
- Mixed-Method Research Enhances Insight Quality: Combining quantitative and qualitative methods offers a more comprehensive view, allowing for both statistical validation and deeper contextual understanding.
- Convergent Parallel Approach: This method collects quantitative and qualitative data simultaneously, enabling researchers to cross-validate findings in real-time, leading to richer insights and faster decision-making.
- Explanatory Sequential Approach: Start with quantitative data to identify trends and patterns, then use qualitative methods to uncover the motivations behind those trends, ensuring a more focused and efficient research process.
- Exploratory Sequential Approach: Begin with qualitative insights to generate hypotheses, followed by quantitative validation, ideal for exploring new markets or complex behaviors where the variables are not well understood.
- Leveraging Multiple Data Collection Methods: Combining CATI, SMS, and online surveys allows researchers to maximize reach, improve response rates, balance cost and speed, and capture diverse feedback from different demographics.
The key to success is not choosing a single methodology but strategically combining different approaches to create a comprehensive research framework. Mixed-method approaches can significantly broaden respondent reach while adding crucial depth to market studies and delivering powerful insights for better impact. While there are many mixed-method approaches, we provide a few specific frameworks with an overview, sample use case, and breakdown of potential methodologies, followed by strategies for mixing data collection methods.
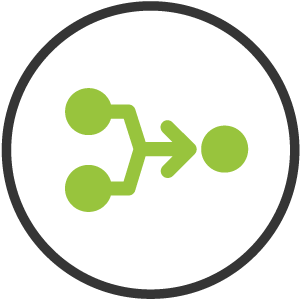
Convergent Parallel
The power of convergent parallel design lies in its approach to simultaneous data collection for comprehensive insights. By concurrently collecting and analyzing quantitative and qualitative data, researchers can uncover rich insights that might remain hidden when using single methodologies. This simultaneous approach creates a dynamic interplay between statistical evidence and human understanding, often revealing unexpected patterns and connections that drive strategic decision-making.
This approach is particularly valuable because it can validate findings in real-time. While quantitative data provides the "what" through statistical significance and trend analysis, qualitative insights illuminate the "why" through rich, contextual understanding. This dual-track approach allows researchers to quickly identify areas where the data converges or diverges, providing opportunities for deeper investigation while the research is still in the field
Use Case
Consider a major consumer packaged goods company launching a new sustainable packaging initiative. Using a convergent parallel design, researchers could simultaneously conduct a large-scale quantitative survey measuring consumer attitudes toward sustainable packaging while running in-depth focus groups exploring emotional connections to sustainability. The quantitative component might utilize online surveys to gather statistical purchase intent and price sensitivity data. At the same time, the qualitative side employs virtual focus groups to understand the deeper motivations behind sustainable purchasing decisions.
This approach proves particularly valuable when you must validate findings across methods or when time constraints require simultaneous data collection. The key is having experienced teams to manage quantitative and qualitative workstreams effectively while ensuring data quality across all methods.
Methodologies to Consider
Quantitative Methodologies
- Large-scale online surveys
- Purchase intent measurement
- Price sensitivity analysis (e.g., Van Westendorp Price Sensitivity Meter)
- Brand tracking metrics
- Product concept testing
- MaxDiff or conjoint analysis for feature prioritization
Qualitative Research Methodologies
- Virtual focus groups
- Online discussion boards
- Digital ethnography
- Social media sentiment analysis
- In-depth interviews (IDIs)
- Video diaries of consumer packaging interactions
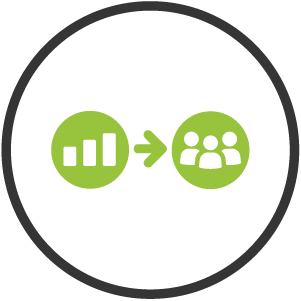
Explanatory Sequential
The explanatory sequential approach represents a strategic two-phase research methodology that begins with rigorous quantitative analysis before diving into qualitative exploration. Researchers collect and analyze quantitative data in the first phase to identify significant patterns, trends, and statistical relationships. These initial findings create a framework of understanding, highlighting areas that require deeper investigation and helping researchers precisely target their subsequent qualitative research.
Building on these quantitative insights, the second phase employs qualitative methodologies to uncover the underlying motivations, emotions, and contextual factors driving the observed trends. This methodical progression from "what" to "why" allows researchers to focus their qualitative investigation on the most statistically significant findings, making the entire research process more efficient and purposeful. The sequential nature of this approach ensures that qualitative research efforts are directed toward the most impactful areas. At the same time, the comprehensive quantitative foundation provides confidence that the deeper insights gained are representative of broader market trends.
Use Case
A financial services company noticed declining engagement with its mobile banking app. Initial quantitative analysis through app usage data and customer satisfaction surveys revealed specific features where engagement dropped significantly. This informed the subsequent qualitative phase, where in-depth interviews with users who showed decreased engagement helped uncover usability issues and unmet needs that weren't apparent in the numerical data alone.
This method combines quantitative research's statistical rigor with qualitative approaches' rich contextual insights. The initial quantitative phase might employ mobile analytics and online surveys, while the follow-up qualitative phase could utilize one-on-one interviews and usability testing sessions.
Methodologies to Consider
First Phase (Quantitative)
- App usage analytics
- Customer satisfaction surveys (CSAT)
- Net Promoter Score (NPS) tracking
- Feature utilization metrics
- User behavior tracking
- Drop-off/abandonment rate analysis
- Session duration metrics
- Transaction completion rate
Second Phase (Qualitative)
- In-depth user interviews
- Usability testing sessions
- User journey mapping
- Think-aloud protocols
- Task completion analysis
- Contextual inquiries
- Critical incident technique interviews
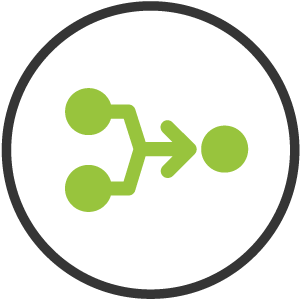
Exploratory Sequential
The exploratory sequential approach inverts the traditional research hierarchy by starting with an in-depth qualitative investigation before moving to quantitative validation. This methodology begins by gathering rich, contextual insights through qualitative research, allowing researchers to understand nuanced behaviors, uncover unexpected patterns, and generate well-informed hypotheses about market dynamics. These initial qualitative findings serve as the foundation for developing more targeted and effective quantitative research instruments, ensuring that subsequent large-scale studies ask the right questions in the right way.
Once these initial insights are gathered, researchers transition to the quantitative phase to test and validate their hypotheses across a broader, more representative sample. This progression from qualitative discovery to quantitative validation combines the best of both worlds: the deep, nuanced understanding of qualitative research with quantitative methods' statistical reliability and generalizability. The approach is particularly valuable when exploring new markets, developing innovative products, or investigating complex consumer behaviors where the key variables and relationships aren't yet well understood.
Use Case
When a healthcare provider wanted to improve their patient experience, they started with in-depth patient interviews and journey mapping sessions to identify pain points and opportunities. These qualitative insights informed the development of a comprehensive quantitative survey that validated the findings across their entire patient population. This approach ensured that the final recommendations were profoundly insightful and statistically valid.
Methodologies to Consider
First Phase (Qualitative)
- In-depth patient interviews
- Journey mapping sessions
- Observational research
- Patient diaries
- Staff interviews
- Shadowing exercises
- Experience mapping workshops
- Service blueprint development
Second Phase (Quantitative)
- Large-scale patient satisfaction survey
- Experience ratings across touchpoints
- Statistical validation of journey pain points
- Demographic analysis
- Wait time metrics
- Service quality measurements
- Correlation analysis of satisfaction drivers
- Regression analysis of key factors
While the previous sections focused on mixed methods research design frameworks (Convergent Parallel, Explanatory Sequential, and Exploratory Sequential), it's equally important to consider how mixing data collection methodologies can enhance research outcomes. Let's now explore how combining different data collection approachesâspecifically CATI, Online, and SMSâcan significantly improve respondent engagement and insight quality.
Mixing CATI, Online, and SMS Can Enhance Your Insights
We all know that consumers are increasingly challenged for their attention, so it is becoming more difficult to reach the right audience efficiently and effectively. Sometimes, relying on a single data collection method can limit response rates, exclude specific demographics, or lead to incomplete insights. Increasingly, researchers are opting to support mixed approaches to data collection to maximize participation, improve representativeness, and deliver budget efficiencies.
1. Expanding Reach and Engagement
Different respondents have different preferences. CATI works well for specific demographics and those who need assistance, as well as studies that require a genuinely representative gen pop sample. Online surveys appeal to consumers who are more comfortable with technology, and while that is undoubtedly the majority of the population, it is not everyone. It also lends itself to individuals who are comfortable expressing themselves, which is also not for everyone. Meanwhile, SMS is perfect for quick, high-response-rate follow-ups and hard-to-find audiences. You reach a broader and more diverse audience by using a mix of these methods.
2. Improving Response Rates and Data Quality
A multi-method approach helps ensure higher response rates. A respondent might ignore an online survey but answer a short SMS follow-up. Others may be more willing to answer a few key questions via CATI before switching to online for a longer survey. Mixing methods increases the chances of survey completion and reduces drop-off rates.
3. Balancing Cost, Speed, and Depth
Each method has its advantages:
- CATI provides in-depth qualitative insights, but is costly.
- Online surveys are cost-effective and scalable.
- SMS surveys deliver quick, real-time responses.
- Using a combination balances cost and efficiency while still capturing rich, high-quality data.
4. Capturing Real-Time and Follow-Up Insights
If a respondent starts a CATI survey but canât finish, an SMS follow-up ensures no data is lost. After an initial short-form response, online surveys allow in-depth exploration of key topics.
Use Case
The research team initially chose CATI surveys, believing they would provide high-quality responses and allow for in-depth customer feedback. Additionally, they wanted to ensure representation from all demographics. Based on profile data on their records, calls were made to citizens who had recently visited the service location.
Challenges Faced
- Low Response Rates â Many customers ignored calls or refused to participate due to survey fatigue.
- High Costs â Each completed interview was expensive due to labor-intensive calling efforts.
- Slow Data Collection â The client needed quick insights, but the CATI process was time-consuming.
Pivot to SMS & Online Surveys
To address these challenges, the research team pivoted to a mixed approach:
- SMS Surveys for Quick, High-Response Engagement
Immediately after a service visit, citizens received a short SMS survey:
"Thank you for visiting us today! How would you rate your experience today? Reply 1-5." If they responded negatively (1-2), they received a follow-up question asking for specific feedback. - Online Surveys for In-Depth Insights
Citizens who completed the SMS survey were invited to a longer online survey via a personalized link. This allowed for open-ended feedback and analysis of pain points.
Outcome & Results
- Response rates increased significantly compared to CATI.
- Cost per response dropped by 50%, making the study more efficient.
- Faster data collectionâkey insights were gathered within days instead of weeks.
- There was more diverse feedback, as younger citizens, who were previously unreachable via CATI, engaged more via SMS and online.
Combining CATI, online, and SMS creates a more inclusive, adaptable, cost-effective research strategy. It ensures you reach the right people, increase response rates, and collect accurate, high-quality dataâall while optimizing costs. Research purists might argue that mixing data collection methods brings biases; however, from a business perspective, maximizing customer participation for feedback is likely a more pressing business issue.
Methodologies to Consider
- CATI
- SMS
- Online Surveys
- In-depth Probing
- Root Cause Analysis
- Barriers and Triggers
- Conversational AI
- Journey mapping sessions
- Experience ratings across touchpoints
- Demographic analysis
- Service quality measurements
The Upside of Mixed-Method Research
Mixed-method approaches offer unparalleled depth and breadth in understanding consumer behavior. By combining different methodologies, researchers can validate findings across multiple data sources, uncover unexpected insights, and develop more nuanced recommendations. This approach gives stakeholders the statistical confidence they need and the rich, contextual understanding that drives meaningful action.
The Downside of Mixed-Methods Research Design
While powerful, mixed-method approaches require significant expertise to execute effectively. They often demand more time, resources, and sophisticated project management capabilities than single-method studies. Additionally, synthesizing disparate data types requires experienced analysts who can integrate findings meaningfully while maintaining methodological rigor.
The key to successful mixed-method research lies not in applying it universally, but in knowing when and how to leverage these approaches effectively. It requires a research partner with deep expertise across multiple methodologies, strong project management capabilities, and the ability to synthesize complex data into actionable insights. When adequately executed, mixed-method research provides a powerful toolkit for understanding consumer behavior and driving business impact.
The most successful research outcomes often stem from carefully constructed methodological frameworks that align with specific research objectives. Having a partner who understands the nuances of different research approaches and can seamlessly integrate multiple methodologies often differentiates between good and exceptional results.